EMIF-PLATFORM
EMIF Platform
EMIF-Platform Project Overview
EMIF has ultimately build an integrated, efficient Information Framework for consistent re-use and exploitation of available patient-level data to support novel research. This will support data discovery, data evaluation and then (re)use.
EMIF-Platform System Overview
EMIF-Platform has developed an IT platform allowing access to multiple, diverse data sources. The EMIF-Platform made this data available for browsing and allows exploitation in multiple ways by the end user. EMIF-Platform has leveraged data on more then 62 Million European adults and children by means of federation of healthcare databases and cohorts from 7 different countries (DK, IT, NL, UK, ES, EE), designed to be representative of the different types of existing data sources (population-based registries, hospital-based databases, cohorts, national registries, biobanks, etc.).
The EMIF Data Catalogue is now available outside of EMIF to bona fide researchers – more information via here.
EMIF Platform EHR Video
This video provides an overview of a service approach via EHR data post-IMI
EMIF Platform Cohort Video
This video provides an overview of a service approach via Cohort data post-IMI
EMIF-Platform Objectives
To achieve EMIF-Platform objectives, the project was divided into eight work packages (WP9-WP16 below). In addition, there are four EMIF-AD work packages and four EMIF-MET work packages to explore.
EMIF-Platform Achievements
Tool Development
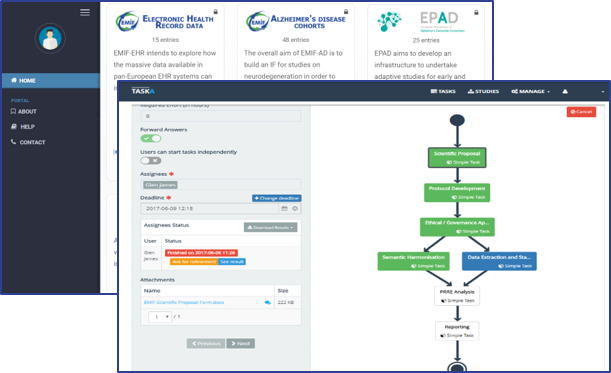
- Key tool developed – EMIF Catalogue as data “shop window” to support the platform architecture, also being utilised by other initiatives (ADVACE, MOCHA, IMI-EPAD, DP-UK)
- Development & integration of TASKA in the EMIF catalogue to manage the workflow.
Common Data Model
EMIF: OMOP-CDM, OHDSI
- Mapped 10 European Databases to the OMOP-CDM
- Contributes to the extension of the CDM and Standardized Vocabularies to accommodate the European data
- Supports the European OHDSI initiative to stimulate adoption of the CDM and collaboration across Europe
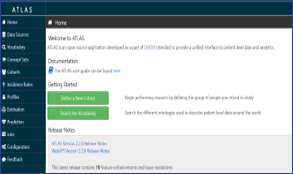
Atlas
- ATLAS tool developed by OHDSI is used to conduct scientific analyses on standardized health data
- EMIF is evaluating the OHDSI tools in the EMIF community and is actively contributing to their further development
Biomarker Discovery
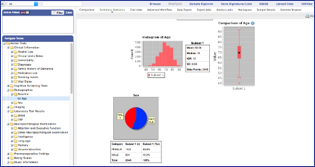
- Raw cohort data integration and analysis via tranSMART and allied bioinformatics tooling development to support biomarker discovery in AD and metabolic disorders
- 3423 subjects from 14 AD cohorts harmonized
- Support of multi-omics data analysis.
EMIF-Platform Tools
WP 9 Framework requirements & evaluation
Carlos Díaz (Synapse) – Peter Egger (GSK)
Synapse (CO-LEAD), GSK (CO-LEAD), EMC, Janssen, UCL, PENTA, EMBL, EUROREC, UAVR, ULEIC, UPF, AP-HP, ARS, STIZON, AUH, GENOMEDICS, BIPS, PEDIANET, UTARTU, UNIMAN, BF, CUSTODIX, Pfizer, SERVIER, Amgen, UCB, MERCK, IDIAP JORDI GOL
GOALS & OBJECTIVES
The objectives are:
- To continuously elucidate user requirements and evaluate the EMIF-Platform as it is progressively developed and deployed.
- For each cycle of the work plan, ensure adequate input from prospective users, especially the present and future research projects, and input from EFPIA participants, as important future users of the platform.
- For each cycle of the work plan, evaluate from the users’ perspective the results of that cycle, feeding it back to the development work packages.
WP 10 Governance, federation, DB fingerprinting, legal context & ethics
Nigel Hughes (Janssen) - TBD (EMC)
EMC (CO-LEAD), JANSSEN (CO-LEAD), SYNAPSE, UCL, PENTA, EMBL, EUROREC, UPF, ARS, STIZON, AUH, GENOMEDICS, BIPS, PEDIANET, UTARTU, UNIMAN, BF, GSK, SERVIER, BI, Amgen, AE. IDIAP Jordi Gol
GOALS & OBJECTIVES
The objectives are to:
- Provide detailed information (the “fingerprint”) of each participating database with respect to population included in that database, the data available in each database, the local data model, the mechanism generating the data, and the ability to address specific re-use issues (such as ability to contact the GP or patients, or inclusion of patients in clinical trials).
- Specify the procedures that will be followed in the governance of the federation, including the required safeguards and criteria for admission.
- Define the different types of users of the EMIF-Platform, the levels of access for each type of user, and the procedures and safeguards to handle permissions.
- Ensure that the project follows ethical principles and conforms to relevant international and national regulations in this regard.
WP 11 Harmonization & semantics
Dipak Kalra (UCL) – Michel Van Speybroeck (Janssen)
UCL (CO-LEAD), JANSSEN (CO-LEAD), EMC, UPF, AP-HP, ARS, STIZON, AUH, GENOMEDICS, BIPS, PEDIANET, UTARTU, UNIMAN, BF, GSK, SERVIER, ROCHE, IDIAP Jordi Gol
GOALS & OBJECTIVES
The objectives are:
- To analyse the harmonisation and semantic needs, and devise a common framework of reference that guides all further interoperability work in the project, including metadata.
- To develop a common data model to be used throughout the EMIF-Platform architecture to represent queries and result sets for aggregate and patient-level data.
- To design and implement services to support specific cross-mappings between terminologies used for clinical/medical terms, medicinal products and units of measurement.
WP 12 Data extraction, benchmarking, aggregation & linkage
Peter Rijnbeek (EMC) – Martijn Schuemie (Janssen)
EMC (CO-LEAD), JANSSEN (CO-LEAD), UCL, UPF, ARS, STIZON, AUH, GENOMEDICS, PEDIANET, UTARTU, UNIMAN, BF, CUSTODIX, GSK, SERVIER, BI, Amgen, ROCHE, IDIAP Jordi Gol
GOALS & OBJECTIVES
The objectives are:
- To develop a common framework for extracting data across different data sources.
- To benchmark the results of the extractions across different data sources with the explicit objective to account for any differences observed.
- To provide tools for linking data between different sources that will allow individuals to be tracked through those data sources, taking into account the transition from childhood to adulthood.
- To aggregate data in order to comply with the privacy and governance rules that control the re-use of data.
WP 13 Analysis, processing & visualization methods and tools
Alvis Brazma (EMBL) – Rudi Verbeeck (Janssen)
EMBL (CO-LEAD), Janssen (CO-LEAD), EMC, UCL, UAVR, ULEIC, UPF, UTARTU, UNIMAN, GSK, SERVIER, PFIZER
GOALS & OBJECTIVES
The objectives are:
- To develop dedicated data analysis algorithms and tools to help exploitation of the data made available through the platfom, leveraging and re-using when possible already existing tools;
- To develop visualisation tools for EHR based on a generalisation of the concept of genome browsers and distributed annotation systems;
- To develop specific analysis tools for broad translational research datasets used in the vertical projects and apply them to data analysis problems arising in the context of such projects.
WP 14 Architecture, solution development, security & privacy technologies
José Luis Oliveira (UAVR) – Philippe Baudoux (UCB)
UAVR (CO-LEAD), UCB (CO-LEAD), EMC, Synapse, UCL, EMBL, EUROREC, ULEIC, UPF, PEDIANET, UNIMAN, CUSTODIX, Janssen, SERVIER, Amgen
GOALS & OBJECTIVES
The objectives are:
- To engineer an ICT infrastructure for the federation of resources, including dynamic support for common data models and variable operation procedures.
- To provide the technical infrastructure for secure, cross-project data exchange.
- To create a development environment for the EMIF-Platform ecosystem, enabling the creation of software such as a clinical information browser, evolving biomedical knowledge bases and Private Remote Research Environments.
WP 15 Use and sustainability models, community building & outreach
Eva Molero (Synapse) – Bart Vannieuwenhuyse (Janssen)
Synapse (CO-LEAD), Janssen (CO-LEAD), EMC, UCL, PENTA, EMBL, EUROREC, UAVR, ULEIC, UPF, AP-HP, ARS, STIZON, AUH, GENOMEDICS, BIPS, PEDIANET, UTARTU, UNIMAN, BF, CUSTODIX, GSK, SERVIER, Amgen, ROCHE, IDIAP Jordi Gol
GOALS & OBJECTIVES
The objectives are:
- To analyze the needs of different key stakeholders (potential users of the platform) with the aim to develop a sustainability model (business model) that serves those needs while being acceptable to data custodians
- To develop and test different sustainability models derived from the analysis above, which ensure the long-term maintenance and operations of the information framework developed during the project.
- Outreach: WP15 also aims at connecting EMIF with other relevant projects (IMI and other) and has the ambition to identify and connect new data sources to the network.
WP 16 Use and sustainability models, community building & outreach
Carlos Díaz (Synapse) – Bart Vannieuwenhuyse (Janssen)
All EMIF partners
GOALS & OBJECTIVES
The objectives are:
The overall objective of this WP is to ensure the successful implementation of this project on a scientific, financial and management level. To achieve this we will focus on 4 main areas :
- Ensure efficient management of scientific activities and financial allocation/reporting, and organisation of project meetings
- Ensure effective communication between partners to support sharing best practice, maximise synergies and prevent duplication
- Designing and implementing dissemination plans and communication strategy
- Managing of Intellectual Property rights and deriving value of foreground information generated
- Ensure appropriate risk management, particularly for joint risks across the whole programme and at the interface of the three topics
EMIF Code of Practice (ECoP)
The EMIF platform can only be used for: assessing the feasibility of a study and conducting research by bona-fide research organisations with the objective of discovering new knowledge intended for the public good and made publicly accessible (i.e., published)
ECoP was developed in order to help ensure that:
- the EMIF Platform and Services are used in ways that comply with legislation, the 2018 European GDPR and policies on data protection
- EMIF upholds best practices in the protection of personal privacy and information governance
- EMIF promotes best practices in the conduct of clinical research using health data, for the public good
Data sources | Data users |
---|---|
will always have autonomy over which data are made accessible and for which types of research | must adhere to the ethical rules and privacy protection policies of each data source |
will always determine ethical acceptability and scientific validity | may only use the data for the specific agreed research purposes |
must be transparent about their data | must acknowledge the sources of the data they have used, and EMIF |
EMIF Catalogue
The key idea of the EMIF Catalogue is to allow researchers to find specific databases aligned with their research purposes, providing a summarized overview of a number of geographically scattered healthcare databases. To accomplish this, the EMIF Catalogue is a flexible web system supported by the Community concept, i.e., a group joining databases and users with the same clinical interests.
Features:
- Community-based access and management
- Database fingerprinting
- Free text and advanced searches
- Suitability assessment (through Jerboa and Achilles)
- Distributed management of groups and data
- Role-Based access control (RBAC)
- Open architecture (plugin-based)
TASKA
TASKA is an innovative platform designed to streamline the creation of modular and easily extendable workflows to manage data extraction and handling general work processes. It is based on Software-as-a-Service approach, making it more versatile and easy to integrate by third party applications.
This platform allows several users to collaborate and interact in the creation and execution of distributed workflows, relying on an easy-to-use interface for managing complex procedures. Features:
- Easy-to-use visual editor
- Task definition, with inputs, outputs, and dependencies
- Questionnaire tasks
- Workflow schemes (templates)
- Running studies (workflows)
- Task assignment and notifications
- Web services that allow the system to operate without any interface
Jerboa Reloaded
Work package 12 (Data extraction, benchmarking, aggregation & linkage) is mainly involved in the important and challenging task to develop the complex scenario when informed consent cannot be obtained. For example, in the case of electronic healthcare records (EHR) collected from general practitioners, access to patent data will often be restricted to only anonymized and aggregated data.
The Jerboa Reloaded extraction tool is developed in the EMIF project to support the data extraction and processing from the EHR databases. It is used in a so-called distributed network design, i.e. it runs de-identification, linkage, analysis and aggregation locally at each data source site. Jerboa runs a script that contains all parameters of a specific study design. This has the advantage that the local analyses are performed in a common, standard way and are not subject to small differences in implementation by local statisticians, and that for each study-only data necessary for that particular study is shared in analytical dataset.
OCTOPUS Private Remote Research Environment
The OCTOPUS infrastructure is used as a prototype for the private remote research environment (PRRE) in WP12. It allows for secured file transfer from and to the data custodians and can be used to collaborate on the aggregated data generated by Jerboa Reloaded.
The OCTOPUS remote research environment is a socio-technological framework that has been developed by Erasmus MC in the past, and has already proven its value in various projects. It stimulates geographically dispersed research groups to collaborate and has resulted in consortia that were engaged in all the phases of the drug safety research. To achieve a successful and sustainable collaboration, database custodians should be more than just data suppliers. As most of the custodians reside in research institutes, analytical tasks should be distributed as well. The main purpose of OCTOPUS is to stimulate such collaborative drug safety research in a secured environment.
In EMIF a dedicated PRRE is currently being developed that is more scalable and further optimized based on the experience with OCTOPUS.
Participant Selection Tool
The Participant Selection Tool allows researchers to get an overview of patient profiles in a given cohort, filtering on a set of predefined key characteristics. The tool has currently been built to provide this capability for AD cohort data sets.
The user interface is designed to minimise the learning curve. Federated data sources are represented in a single tabular or graphical view. Filtering on categorical, date or continuous key variables is possible and the result is a count of the number of matching patients across the different cohorts.
The basis of the tool is the ‘Knowledge Object’. These ‘knowledge objects’ are ontologies to which the different data sources (in this cases cohort data) can be mapped. The use of semantic technology offers the required flexibility as the ontology develops or as additional data sources are added.
The tool can accommodate different projects (e.g. a single data sources can be participating in one or more projects) or additional characteristics can be added for a given project. The same framework will also be used to develop additional tools, ultimately enabling an integrated data pipeline for cohort data.
Virtual Selection Tool (VST)
VST provides the researcher with an overview of available variables (counts, not values), followed by data access request to the selected cohort owners.
TranSMART
TranSMART is a knowledge management application built from open source components to investigate correlations between genetic and phenotypic (clinical) data to aid in predictive biomarker discovery.
It consists of a web-based graphical data mining application that connects to a server based data warehouse. TranSMART was made open source in 2012. The tranSMART Foundation was established in 2013 to provide governance and coordination for new developments. Important contributions have come from IMI eTRIKS, CTMM TraIT, Pfizer, Sanofi and Janssen, amongst others.
TranSMART can combine clinical and high dimensional data, such as gene expression, single nucleotide polymor-phisms (SNPs), Rules-based medicine (RBM), genome-wide associations studies (GWAS), copy number variations (CNV), etc. In EMIF, the main emphasis has been on harmonized clinical data. High dimensional data is expected from e.g. the EMIF-AD 1000 samples cohort.
TranSMART allows easy generation of queries by phenotypes, genotypes, or a combination. Study groups can be formed ad hoc to generate summary statistics or for hypothesis testing. Several advanced analysis pipelines are built in, such as boxplots with ANOVA, scatter-plots with linear regression, Kaplan-Meier plots with survival analysis, etc. GenePattern functionality is available for gene expression or proteomic analysis. Subjects from individual cohorts can be pooled in a virtual cross trial cohort for a unified data analysis.
Multi-omics Research Environment (MORE)
Cloud computing provides users with a number of benefits: reduction of computational costs, universal access, up to date software, choice of applications, flexibility. There are many cloud platforms that Research projects can choose from: Amazon Web Services, OpenStack, VMWare, Google Cloud, etc. MORE is a transferrable solution between different cloud platforms and has specialised components for clinical and –omics data analysis. In addition, MORE implies a flexible architecture that allows adding new tools and pipelines upon request.
The following pipelines and tools are currently available in MORE (version 2):
- tranSMART for clinical data analysis;
- R Cloud for R parallel computing and R specific analysis;
- iRAP pipeline adapted for the Docker cluster to analyse transcriptomics sequencing data;
- NGSeasy pipeline adapted for the Docker cluster to analyse genomics sequencing data;
- MZmine2 adapted for the Docker cluster to analyse proteomics and metabolomics LC-MS data;
- Sequence Imp pipeline adapted for the Docker cluster to analyse microRNA sequencing data.
The tranSMART, Docker cluster and R Cloud are connected and use a shared file system. The Docker cluster and R Cloud benefit from the scalability of cluster computing usage – multiple VMs, job queues and task scheduler. New resources are added when needed.
MORE is an open-source project and the code is publicly available at: https://github.com/olgamelnichuk/ansible-vcloud. The EMIF instance of MORE is available at EMBL-EBI Embassy Cloud and can be accessible upon a request by EMIF users. This instance is used for EMIF-AD biomarker discovery.
Knowledge Object Framework
Michel van Speybroeck, James Cunningham, et al. Janssen & UNIMAN
The knowledge object framework consists of a number of components that support the harmonization effort of clinical data. A knowledge object is a semantic representation of a clinical variable and contains descriptive metadata, executable rules that specify the relations (mapping) to other knowledge objects, and the actual data. A local knowledge object is the representation of a source variable and contains the raw data. A global knowledge object defines a harmonized, cross trial variable and serves as a mapping target for local knowledge objects. Several levels of derived knowledge objects are possible, thus creating a dependency graph.
The main goal of knowledge objects is to make the data harmonization process more efficient by specifying the minimal information to understand a clinical measurement and its mapping to a harmonized variable. That information is owned and maintained by the local data source or the research community. By specifying metadata and mapping rules using semantic web technology a reasoner can be used to perform the actual data extraction and harmonization. Security restrictions are also defined on local variables and automatically propagated to global knowledge objects.
The knowledge object framework consists of the following technical components:
- A central knowledge object library that contains the definitions of core classes, properties and the global knowledge objects.
- A local ontology at each data source, developed using tools such as Protégé (for definition of local concepts) and Pentaho (for specifying mapping rules and connections to local data sources).
- Executing the mapping rules results in a local dataset, harmonized to the global standard and stored in a Stardog triple store. The Stardog sparql API makes the data available to federated queries from outflow tools such as the Participant selection Tool or the Variable Selection Tool.
OMOP CDM/OHDSI Tools
OHDSI tools (e.g. ACHILLES) provides researcher with an overview of patient profiles in a given cohort, with filtering for a limited set of pre-agreed characteristics.
- Mapped 10 European Databases to the OMOP-CDM
- Contributes to the extension of the CDM and Standardized Vocabularies to accommodate the European data
- Supports the European OHDSI initiative to stimulate adoption of the CDM and collaboration across Europe
Atlas
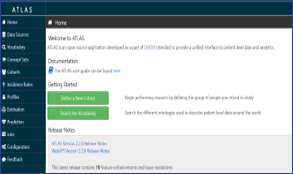
- ATLAS tool developed by OHDSI is used to conduct scientific analyses on standardized health data
- EMIF is evaluating the OHDSI tools in the EMIF community and is actively contributing to their further development
Workflow Management
Workflow management ensures integrity of process between researcher and data sources; management of tasks and process steps.
Workflow Managment
Workflow management ensures integrity of process between researcher and data sources; management of tasks and process steps.
Switchbox
Switchbox provides a single interface for cohort owners, but multiple harmonised outputs for tools.
Corhort Selection Tool (CST)
CST provides the researcher with an overview of the potential cohort data, availability, and suitability.